Okay, back to CUDA and GPU acceleration. Today we're going to work through the example I gave in the previous introduction, but in more detail. (Series starts here, next post here)
A reminder; in the CUDA world the CPU is called "Host", and the GPU is called "Device".
To recap, here's our vanilla C program, cleaned up a bit:
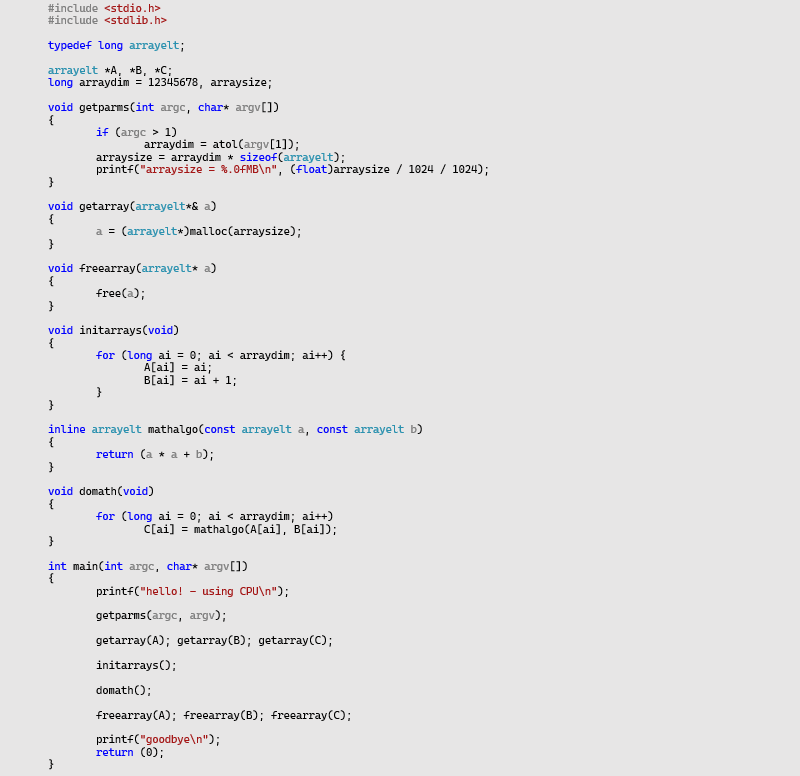
As you can see this is plain-old C code, nothing fancy. At the bottom we have the main() function which says "hello", gets parameters, allocates three arrays named A, B, and C, initiatizes the arrays, does the math (computes C = A2+B for every array element), and then frees the arrays.
The getparms() function parses a single parameter, the size of the arrays.
If we save this as hello.cpp (yes, that's a link to the source please download), then compile, link, and run it, we get something like this:

So yay. But since we want to measure performance, let's make a slight change to give us timings. Here is hello1.cpp:
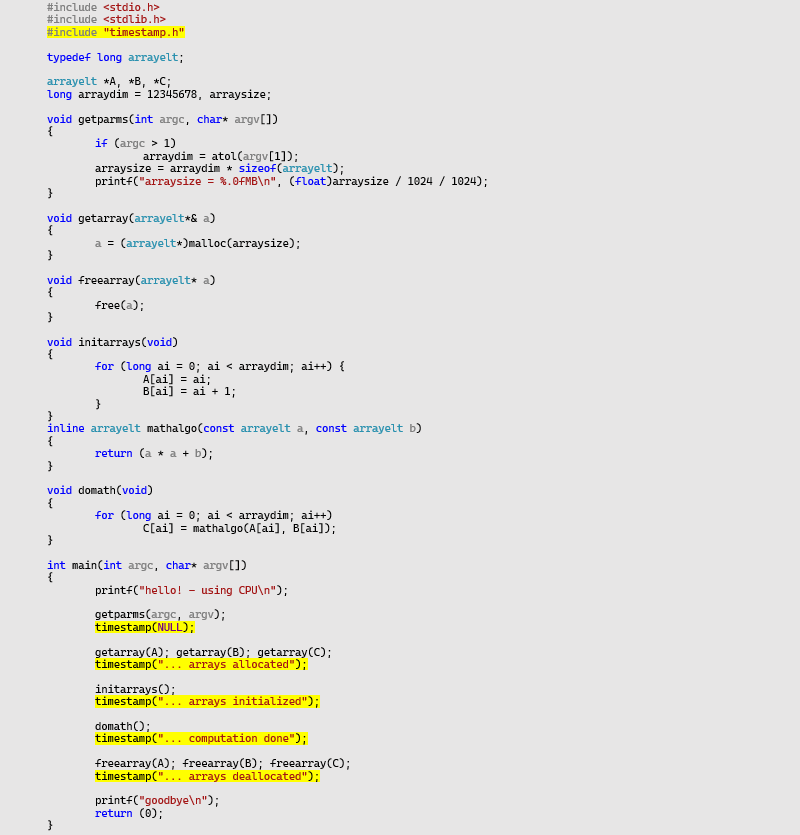
The changes from hello0 are highlighted; there's a new header timestamp.h which defines a timestamp() function which displays timestamps, and a few calls to timestamp() interspersed into the processing. If we compile and run hello1, it looks like this:
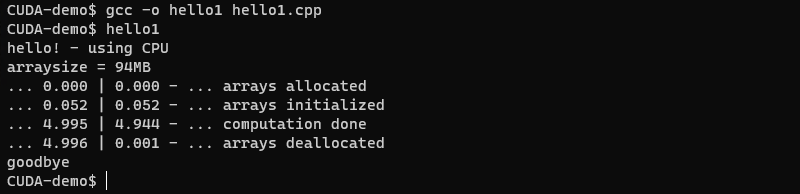
The timestamp() function displays the cummulative time and time interval in seconds. So the array allocation took 0s, the initialization .05s, and the computation 4.94s, for an overall time of about 5s. That's for the default array size of 12,345,678. Let's add another order of magnitude, by specifying 123,456,789 as the array size parameter:
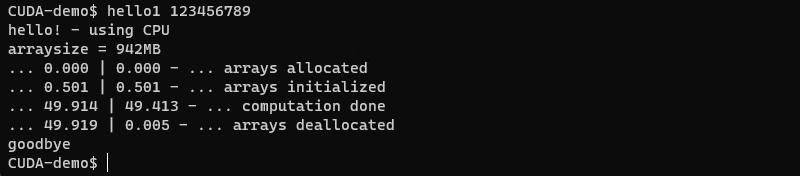
So now the computation took about 50s. That's a long time. But the whole process ran on the CPU, and it ran serially under a single thread. Let's make it faster!
Okay, so now we convert this into our first CUDA program, hello2.cu (note it is .cu now instead of .cpp):
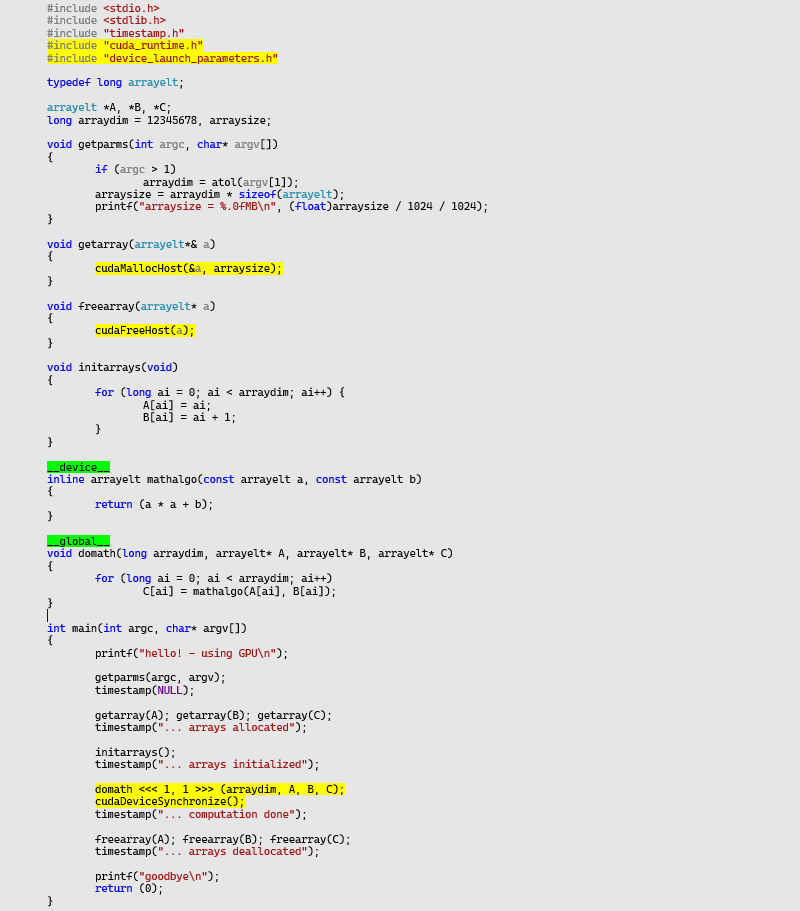
As before the changes are highlighted. At the top, we've added two more headers which contain CUDA runtime library declarations.
The array storage allocation was changed from malloc() to cudaMallocHost(). We'll talk about memory in more detail a bit later, but this function allocates storage which is addressable by both the Host and the Device. Correspondingly, we changed free() into cudaFreeHost().
The mathalgo() function is now scoped with __device__ to indicate it should be implemented on the device, not the host (green highlighting). And the domath() function is scoped with __global__ to indicate it should be implemented on the device, but callable from the host (also green). Note that the other functions could be scoped with __host__ to indicate they run on the host, but this is the default so we didn't have to do this.
The domath() function call in main() was changed; we added two parameters enclosed by triple-angle brackets. This is a CUDA extension to C which designates a call from the Host to the Device (from a __host__ scoped function to a __global__ scoped function). This call actually spawns one more threads to run in parallel on the device, as we shall see, an action that is referred to as invoking a kernel, where "kernel" is used to describe the code and threads which will run on the Device.
And the domath() kernel invocation is followed by a call to cudaDeviceSynchronize(). This causes the Host to wait for the Device thread(s) to complete.
Okay, so that was cool, let's compile hello2.cu (with nvcc instead of gcc), and run it:
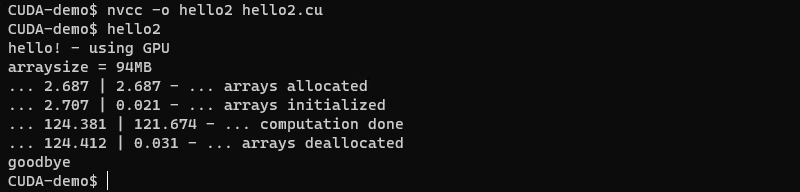
Well yay, it ran! But ... boo, it took forever - nearly two minutes! Using a GPU is supposed to be faster, what happened?
Well it turns out that we ran a single thread. Here's a picture to show what hello2 does:
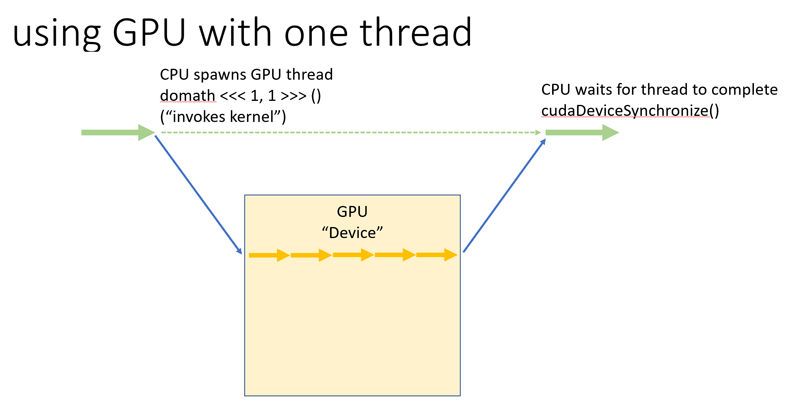
We invoked the kernel to run a single thread on the GPU. Any one thread on the GPU is way slower than a thread on the CPU, not to mention the overhead of starting the process and waiting for it to finish.
So what to do? We want to run a bunch of threads in parallel, like this:
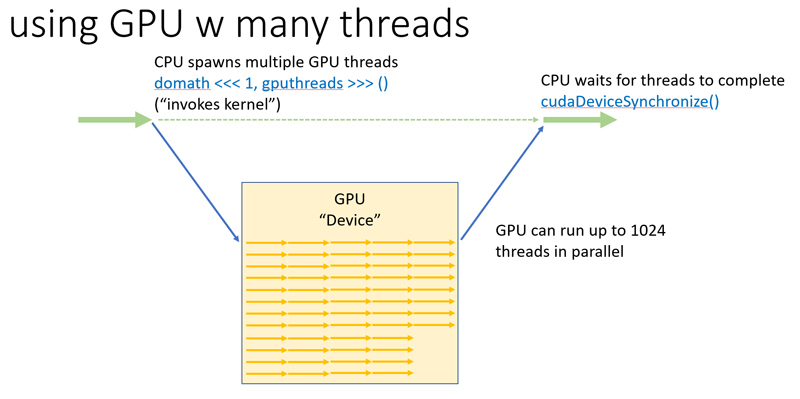
So let's make a simple change to run multiple threads in parallel; here's hello3.cu:
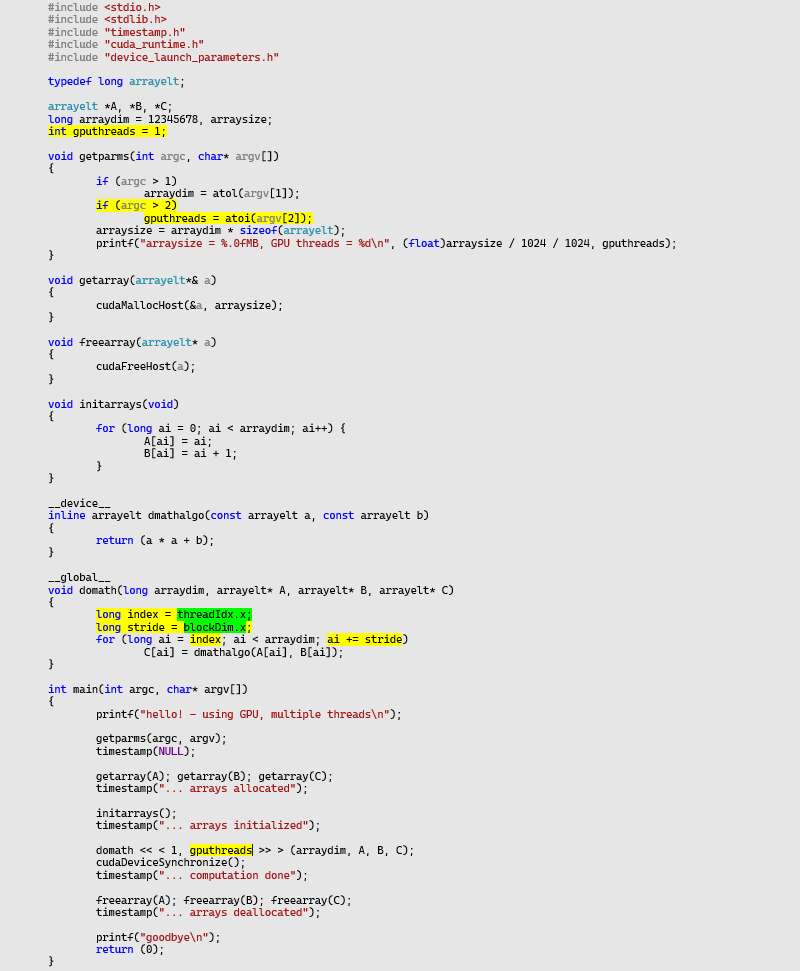
The highlighting shows the changes. First, we've added a new parameter to specify the number of GPU threads.
In the main() function the kernal invokation of domath() has been changed, we've specified the number of threads as one of the parameters in the triple-angle brackets. (We'll talk about the other parameter soon.)
And we've changed the loop in domath(). We don't want to iterate over the whole arrays in each thread, that would defeat the purpose. We want each thread to process a different piece of the arrays. The global values threadIdx and blockDim are available in __global__ and __device__ code, and can be used to give the current thread's index and total number of threads. Each thread therefore starts at a different place in the arrays, and iterates though using the number of threads (the "stride").
Let's say we have 100 threads. The first thread starts at array element 0, and then processes 100, 200, 300, etc. The second thread starts at 1, then does 101, 201, 301, etc. And so on through thread 100, which starts at 99, then does 199, 299, 399, etc. Each of the 100 threads processes 1/100th of the array, which is what we want.
Great, let's compile and run hello3 to see how this works:
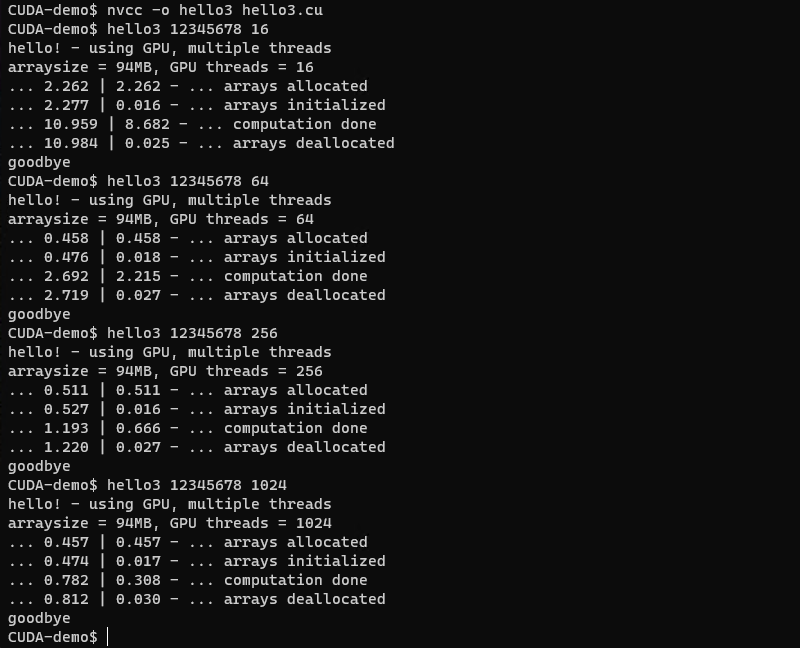
Yippee. Here we have a few different runs of hello3, with different numbers of threads, specified as the second parameter.
Remember that hello1 ran on the CPU in 5s? And hello2 ran using the GPU single threaded and took 120s. With 16 threads, hello3 took 11s, with 64 threads, 2.7s (already better than the CPU), with 256 threads, 1.2s, and with 1,024 threads, .8s!
Let's go back and try that test we did above, where we added another order of magnitude to hello1, and try it with hello3:
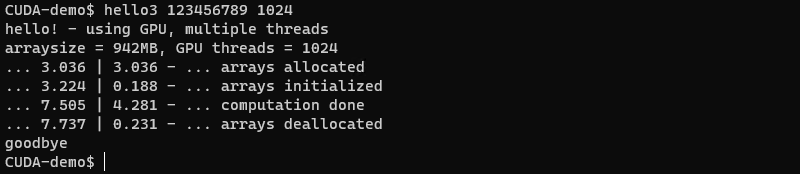
Excellent! This took about 50s on the CPU, and just 7.7s with GPU acceleration.
However there is more we can do to go even faster - stay tuned for the next installment of this series...
|